On last week’s edition of TradersMagazine’s Tech Tuesday, I read the interview with Mike O’Rourke, Head of AI and Emerging Technology at Nasdaq. Packed with great insight, I found his forward-thinking stance on AI particularly interesting because, as early as 2016, he identified its potential and proactively built a center of excellence for AI at Nasdaq. This led me to further understand Generative AI’s role in the current context of the US equity markets and the broader implications of using it for market and trade surveillance.
- How evolved are AI systems today?
- What are the current use cases, and how much human involvement is important?
As I explored these questions, I realized the answers were as complex as the technology itself. Let’s begin by exploring current AI use cases for trade surveillance. Generative AI (GenAI) involves AI systems that create new content, such as text, images, and code. Trained on large datasets, these models learn to generate outputs that mimic human-like content and are used in applications like trade surveillance to automate and enhance monitoring tasks.
Streamlining and Triaging Alerts
Traditionally, surveillance systems have relied heavily on rule-based algorithms that have struggled to keep pace with sophisticated trading strategies and manipulative tactics. Gen AI has the potential to change this dynamic by employing advanced machine learning models to detect anomalies and patterns indicative of market abuse, such as unusual trading volumes or suspicious price fluctuations.
Surveillance teams often review hundreds of alerts daily and face significant challenges distinguishing between false positives and activities that warrant a deeper investigation. Gen AI can streamline this process by swiftly analyzing and highlighting pertinent information and key details from various sources, including trusted news outlets and corporate filings. This capability allows for rapid triaging of alerts, enabling investigators to determine which cases require further analysis.
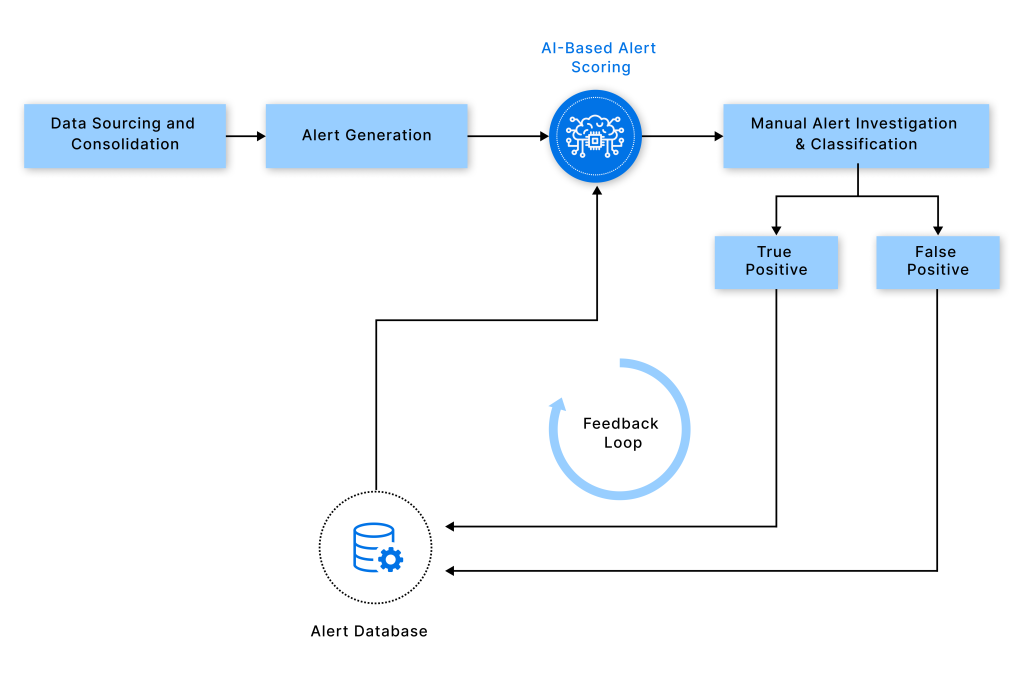
Surveillance analysts who participated in a proof-of-concept test estimated that the new AI features led to a 33% average reduction in investigation time – while simultaneously leading to improved overall outcomes.
Just triaging the initial batch of alerts can be highly time-consuming, so we set out to streamline this process by combining our expertise in the space with the best in generative AI.”, Tony Sio, Head of Regulatory Strategy and Innovation at Nasdaq.
Enhancing Regulatory Compliance
Gen AI acts as a virtual expert, capable of parsing extensive regulatory texts and other long-form documents to provide concise, actionable summaries. This allows compliance officers to stay abreast of the latest regulatory changes and ensure alignment with SEC guidelines and other compliance frameworks. AI-driven systems can automatically check trading codes and algorithms for compliance, flag potential misalignments, and suggest modifications to adhere to the latest regulatory standards. This AI capability extends to comparing different regulatory frameworks and company operating procedures, ensuring alignment, and identifying discrepancies. Gen AI can be useful in migrating old analytical models from legacy programming languages like SAS or COBOL to more modern ones like Python. This technology automates the monitoring of model performance across trading platforms, generating alerts if the trading activities fall outside established tolerance levels. It also assists in drafting comprehensive model documentation and validation reports, which are crucial for regulatory compliance and internal audits.
Enabling Climate Risk Assessment
Although more indirectly related to trade surveillance, Gen AI can be useful in assessing climate risks that might impact equity markets. It can automate the data collection for counterparty risk assessments and generate early-warning signals based on environmental trigger events. This capability is increasingly important as the financial sector grows more attuned to environmental, social, and governance (ESG) factors.
The Human Aspect of AI for Surveillance: A Symbiotic Relationship
There will always be a human aspect to surveillance to ensure robust systems and controls are in place and to provide an additional layer of protection for trading systems. It’s a symbiotic relationship; by giving the analysts better AI tools, they are able to use new techniques or incorporate new datasets that they wouldn’t have had time to previously, which we can then use AI tools to make even more efficient.
In Summary
With legacy systems falling short and increased regulatory scrutiny, there is a need to rethink tool-based trade surveillance. AI-based systems offer new opportunities for improved trade surveillance practices. This has a twofold benefit—it benefits not just the financial institutions deploying surveillance but also regulators. However, as with any new technology, the transition or integration needs to happen in a structured manner. While understanding the underlying mechanisms, it’s important to chalk out well-defined steps while implementing AI tools for trade surveillance. Transparent information transmission between compliance officers and regulators and proper training allowing proper reporting are other areas to focus on.
By maintaining a dialogue between regulators, technologists, and financial experts, the capital markets industry can foster an environment where AI thrives as a surveillance tool and operates within a framework that ensures its decisions are fair, accountable, and transparent. This nuanced approach will be crucial in harnessing AI’s full potential for trading systems.