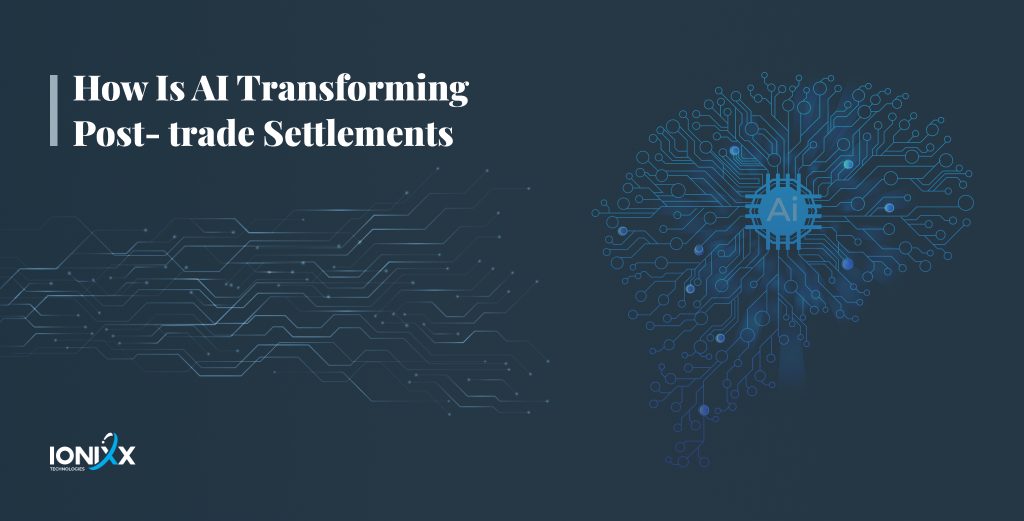
The post-trade segment of the securities market has remained heavily regulated with decades of legacy processes and systems driving its operations. However, currently, it’s at an interesting inflection point, undergoing a paradigm transformation.
Warming up to leveraging the capabilities of newer technologies and APIs, the industry is exploring how Artificial Intelligence (AI) will fit in. The reasons are aplenty.
For one, staggering trade failure rates within post-trade settlement systems remain a top concern.
A 2019 Depository Trust & Clearing Corporation (DTCC) report estimates that even a negligible 2% trade settlement failure rate could potentially result in costs and losses of over $3 billion.
DTCC Report
There is a 3% failure rate among corporate bonds and sovereign debt markets, while equities reach up to a staggering 6%.
European Securities and Markets Authority
AI use cases across securities markets are not entirely new. Several asset managers have been dabbling with AI in investment strategies, risk management, and compliance.
Typically, AI has been useful in allowing traders, brokers, and financial institutions to optimize market orders during execution and streamline post-trade workflows. This has been a key contributor in minimizing settlement failures.
AI is also considered significant for its role in information access and sourcing, given how data management and integration are central to successful trade settlement.
In this article, we explore the promise of AI for post-trade settlements; and how it adds layers of automation to minimize instances of trade failures, thus offering a competitive edge to broker-dealers.
Why AI for Post-trade?
According to a SIFMA research, in its Capital Markets Fact Book 2023, in 2022, the average daily trading volume for equities was 11.9 billion shares, an increase of 4.1% Y/Y.
Amidst this data and volume surge, the post-trade landscape continues to evolve with rising costs and margin pressures, greater risk and compliance requirements, and increased regulatory interventions. Now is the time to re-assess existing post-trade systems for their efficiency in handling growing trade volumes.
Understanding Trade Settlement
For a trade to be executed successfully, several things need to fall in place. Multiple intermediaries, continuous exchange of messages between these intermediaries, and higher transaction costs make this quite a challenge.
Let’s break down the entire trading lifecycle step by step.
- The DTCC provides clearing, settlement, and information services for equities and other securities traded on US financial markets.
- Fulfilling the clearing role is the National Securities Clearing Corporation (NSCC), through a process called multilateral done via a Continuous Net Settlement (CNS) system. This process helps reduce the number of payments and securities that are exchanged, thus reducing counterparty risk.
- The Depository Trust Company (DTC) maintains a centralized store of stock ownership records.
- If a participant fails to settle with the DTC, the DTC may cease to act for that participant and terminate their membership and are immediately notified to the US Securities and Exchange Commission (SEC).
- Clearing participants include brokers, broker-dealers, banks, and insurance and investment companies.
What Makes A Successful Trade?
Each trading day, stock exchanges electronically transmit details of trades that have taken place.
For a trade that is executed on day T, NSCC’s guarantee of settlement begins at midnight between T+1 and T+2, at which time NSCC steps in the middle of the trade and assumes the role of counterparty for both the buyer and seller.
NSCC multilaterally nets trades by stock and on T+2 notifies participants of their net positions in each stock (net long or net short) due to be settled, as well as summaries of all their trades.
Here is a step-by-step rundown:
- NSCC nets trades that are due to settle on T+2 as well as open positions that were due to settle previously, such as positions resulting from fails.
- On the second business day following the trade (T+2) instructions are sent to the DTC containing net securities positions to be settled.
- The DTC makes the transfers of stock and cash.
- An algorithm run by the NSCC determines which of the participants with long positions (participants that are owed stock by the NSCC) due to be settled that day will receive stock.
- If no participants fail to deliver on their short positions, i.e., the DTC is able to transfer all the stock owed by participants to the NSCC account, and there are no open failed positions from previous days, then all participants receive the stock they are owed by the NSCC.
When participants fail to deliver (FTD) stock, the NSCC receives less stock than it owes and consequently may not be able to deliver stock to all participants with long positions. This in turn results in what we call a failed trade.
Consequences of Trade Settlement Failures
Failures to Deliver
The short shows the value of U.S. Treasury securities that were not delivered to fulfill a trade contract.
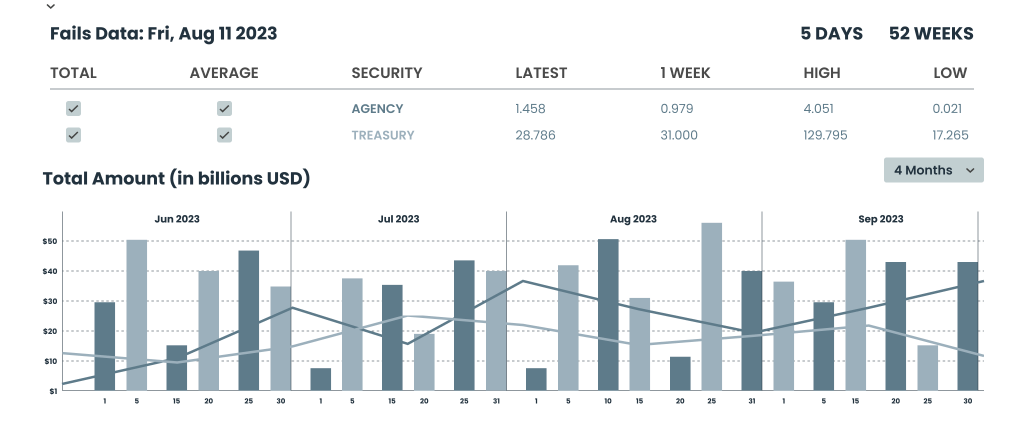
“Failures-to-deliver” (FTDs) occur when either sellers fail to deliver or buyers fail to receive securities in time to settle a trade. When participants fail to deliver stock, the NSCC receives less stock than it owes and consequently may not be able to deliver stock to all participants with long positions.
What Happens When FTD Occurs?
If a participant is unable to deliver on their net short position (stock owed to the NSCC), the position is called a “fail to deliver” and that short position remains open.
The long positions for which the NSCC is unable to deliver stock remain open long positions called “fail to receive” (FTR). This can be thought of as an IOU from the NSCC to the participant with the long position and similarly the FTD can be thought of as an IOU from the participant with the short position to the NSCC.
Let’s assume that a firm fails on a $50 million position on which it is owed $50.1 million. If the Target Fed funds rate the previous day was 1%, then the fails charge will be 2% per year and it will be applied to the $50.1 million total value of the trade. This will result in a charge for the firm of $2,783.33 for that day.
This unfortunate reality is underscored by recent data from the European Central Bank in the 2022 Target2-Securities annual report, which showed that the trade fail rate increased by 0.5% over that of 2018 – where the average fail rate was 2.61% and 3.11% in 2019.
Here is a quick snapshot of the variations in T2S settlement rates:
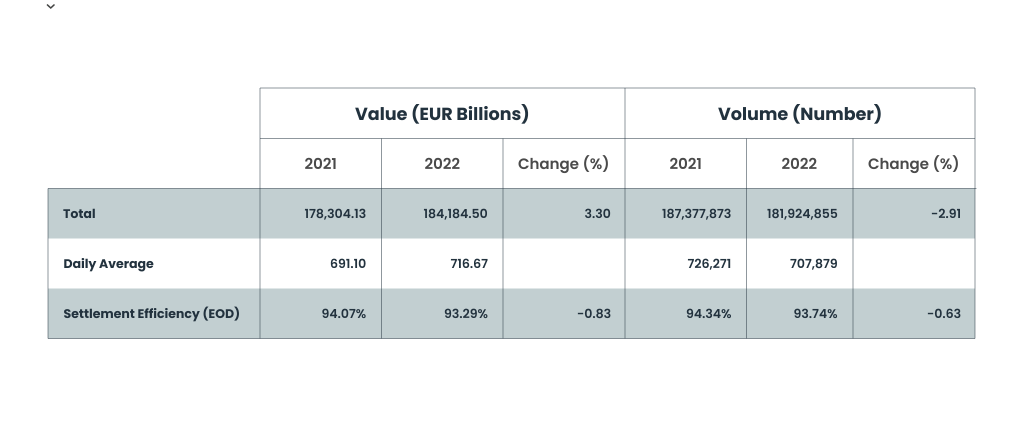
Where Do Current Processes Fall Short?
First up, manual trading processes are often capable of generating unnecessary errors, causing delays in trade confirmation, matching, and affirmation before the settlement date. This can cause inaccurate or stale settlements, leading to trade fails and causing a new set of discrepancies between buyers and sellers. Broker-dealers can get caught in a rut as they try to mitigate errors, spending copious amounts of time on erroneous exceptions. This can have a domino effect across the entire post-trade process. Additionally, low-quality data because of batch processing can lead to fragmented data management, exposing process silos and inefficiencies across the entire post-trade process.
How Can AI be Leveraged to Handle Trade Failures
Predicting And Mitigating Trade Fails to Delivery
AI can play a significant role in predicting and mitigating trade fails by leveraging historical data, pattern recognition, and automation, such as alerting investment managers prior to the settlement date about insufficient securities.
The model’s output is the probability of a trade failure of a security (ISIN) for a settlement date. Based on the probability, broker-dealer operation leaders can identify a group of trades that can potentially fail and involve counterparties to take action, such as addressing a liquidity shortfall. This early alert to counterparties will smoothen trade settlement processing on the settlement date.
AI and Predictive Analytics
AI and Predictive Analytics can be used to identify patterns that may lead to FTD incidents. Predictive models can assess historical data to provide early warnings of potential FTD risks. Using various data-driven methods, including machine learning and statistical modeling, the probability of trade settlement failure can be predicted By analyzing Trade booking and enrichment, inventory management, historical trade data, market conditions, counterparty information, and other relevant factors, you can build predictive models that estimate the likelihood of a trade failing to settle on the scheduled settlement date.
Trade booking | Position Management | Market maker global business and market infrastructure |
Suspended Settlement Accounts/Counterparties | Complexities in settlement processing, cross-border trades | Issuer actions: Buybacks, exchange offers |
Mismatches product information | Underlying securities being blocked as colleterial to meet funding requirements | Corporate reorganizations: perverse splits, bankruptcies |
Lack of discrepancies in trade confirmation | Shortage of securities in cash market | Regulations |
Awaiting trade modifications and corrections | Naked short selling |
Regression Analysis for Predicting Failures
The statistical method of regression can be applied to predict trade settlement fails. Logistic regression is a form of regression where the dependent variable is dichotomous(binary) and the independent variables (influencing factors) can be either continuous or categorical. This methodology is widely used in the financial services industry. In predicting the probability of trade settlement failure, it would be prudent to adopt multinomial logistic regression for greater efficiency in post-trade operations to address trade settlement failures preemptively.
Initially, predicting the probability of failure will be based on historic settlement failure trends, which when fed into a machine learning algorithm will predict the baseline probability of a failure. A feedback loop, such as pattern analysis, will feed data, such as settlement fails and their associated reasons, to refine the algorithm. With iterations, the predictions will better reflect reality.
A detailed analysis of failure reasons can provide insights on the corrective or remedial action to be taken in processes, people, technology, or a combination thereof, thereby improving prediction probability.
The algorithm, for example, may predict that a set of trades of a security are expected to fail on a given settlement date, due to the following reasons (or a combination thereof):
- Security account of the deliverer is “inactive.”
- Insufficient inventory in the deliverer account even after considering projected inflows.
- Impending corporate action (such as a buyback) absorbs liquidity from the deliverer.
Teams can initiate remedial action by alerting the delivering counterparty upfront (prior to settlement date) about the potential of a trade failure along with the corresponding reasons.
These predictions and subsequent proactive measures will help clients in many ways such as reducing the cycle time to resolve trade settlement failures, decreasing time spent firefighting among market participants on trade failures, easing administrative burdens and enabling better deployment of human resources. We project that businesses can reduce the overall number of failed trades by about 40% and ensure teams spend less time on unproblematic trades and instead focus on monitoring those more likely to fail. The accurate prediction of settlement fails will greatly help mitigate or reduce penalties arising out of such failures or violations of regulatory norms.
Next Steps in A Nutshell
Organizations need to clearly identify which areas within post-trade need redressal, define benefits, identify right data, invest in acquiring the requisite skills, and overcome functional silos to enable AI implementation.
They will need to focus on a holistic program management approach, especially in the wake of T+1, after overcoming the initial resistance to change, to be able to see the benefits of AI at scale. Since organizations have limited bandwidth and budgets, implementation planning is a key step, and pilot projects based on identified use cases can be a good litmus test.
With greater collaboration among regulators and market players, the need for standardization and transparency in the capital market value chain is getting more pronounced.
Ionixx And Our AI Capabilities
AI opens up several avenues for brokerage firms to modernize their post-trade processing systems. If deployed methodically, AI can:
- Help transition from proprietary legacy technology to consolidate and streamline data processes.
- Leverage new open-source analytics tools to enable flexibility and scalability.
- Automate manual processes through real-time data processing and simplify cross-asset, cross-product views of the business.
At Ionixx, we help unlock the value of data analytics and AI to ensure a high-quality, consolidated, and integrated post-trade processing and settlement system. Through this, we facilitate firms to be in a better position with respect to the deployment of capital, have a more accurate view of risk and failure, and stay on top of cost control – through consolidation, standardization, and removal of duplicated processes and data.
To speak to our securities expert team and domain consultants, please get in touch.