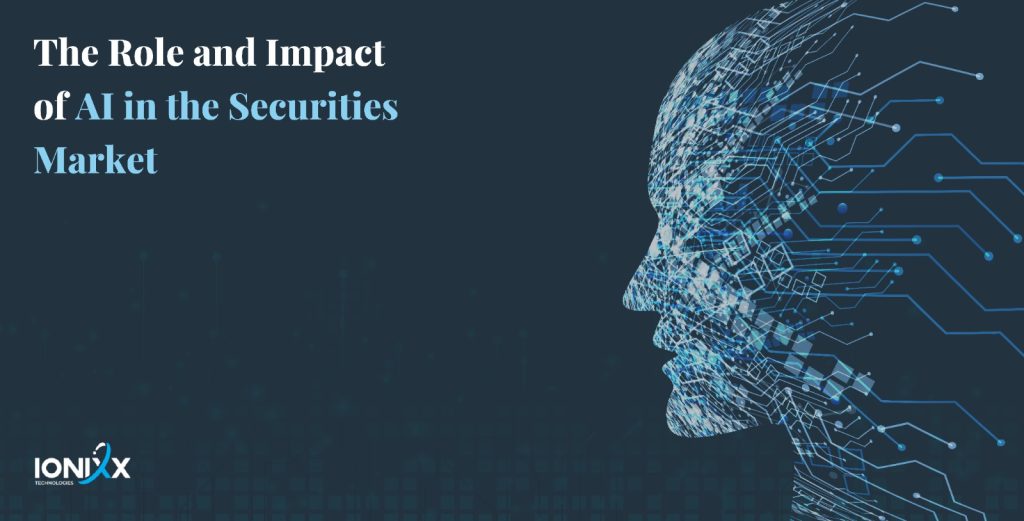
A March 2023 IDC report forecasts worldwide spending on AI systems expected to surpass $300 billion in 2026.
Can AI catalyze change and innovation offering significant value to US security markets?
For starters, it’s interesting to see how DTCC believes that the scope for innovation with AI is promising, and is a crucial changemaker. From expediting settlement times through advanced straight-through processing, and enabling process improvements in the front office, to automating and streamlining post-trade operations, AI-driven technologies are poised to address many of the industry’s challenges.
In this article, we scratch beyond the surface and deep dive to analyze the key value propositions that AI can bring to the securities market.
We explore how AI is reshaping both pre-trade and post-trade activities and its pivotal role in the transition from a T+2 to a T+1 settlement cycle.
Why Is AI A Game-changer for The Securities Landscape?
High-frequency trading (HFT), which heavily relies on algorithms and data, accounts for approximately 50% of the equity trades in the US.
Springer Research
For one, growing volumes of data from multiple sources and the proliferation of algorithmic trading call for a need to adopt efficient data processing systems. AI makes for a great contender in this context given how AI-driven algorithms can help identify trends, patterns, and potential risk factors across the entire trading lifecycle.
By 2030, banks and asset managers are expected to save $1 trillion by implementing AI models for their business operations.
Business Insider ReportTwo, policymakers, regulators, and supervisors around the globe have focused their innovation and attention on considering AI techniques for risk and regulatory management. Christopher Nardo, DTCC Director of Product Management, in his recent article evaluates
AI Impacting Capital Markets
The following table lists AI tools and technologies with their distinct capabilities and the benefits they could potentially bring to capital markets.
AI-driven Technologies | Definition | Impact on Capital Markets | Benefits |
Machine Learning (ML) | A subset of AI that focuses on training algorithms to learn from data and make predictions or take actions without being explicitly programmed | ML enables faster and more accurate decision-making for broker-dealers in capital markets, as it quickly analyzes vast amounts of financial data to identify patterns and trends. | Enhanced prediction accuracy Improved risk assessment Better trading strategies |
Supervised ML | An ML approach where the algorithm learns from a labeled dataset to make predictions or decisions | By using historical data with labeled outcomes, Supervised ML algorithms can make accurate predictions and aid in risk assessment in capital markets. | Accurate forecasting and prediction, Anomalies and fraud detection, Improved portfolio management |
Unsupervised ML | Unsupervised Machine Learning involves training algorithms on unlabeled data to discover patterns or relationships in data. | Unsupervised ML helps identify patterns and relationships in vast datasets without predefined labels, aiding in market segmentation and anomaly detection. | Efficient market clustering, Enhanced customer segmentation, Streamlined anomaly detection |
Reinforcement Learning | Reinforcement Learning focuses on training algorithms through trial and error and rewarding or punishing actions based on feedback | Reinforcement Learning can aid in optimizing trading strategies and portfolio allocations by adapting to changing market conditions and | Improved trading decision-making, Effective risk management, Dynamic personalization |
Deep Learning | Deep Learning is a subset of ML that uses artificial neural networks to imitate human-like thought processes. | Deep Learning models can analyze complex financial data and predict market trends, enabling better investment decision-making. | Accurate sentiment analysis, Enhanced fraud detection, Improved trade execution, and automation. |
Natural Language Processing (NLP) | Natural Language Processing (NLP) is a branch of AI that focuses on enabling computers to understand and interpret human language. | NLP enables computers to understand and interpret human language, thus allowing the automation of tasks like customer inquiries and news analysis. | Efficient news sentiment analysis, Automated reports generation, |
Computer Vision | Computer Vision uses AI to enable machines to analyze and interpret visual information. | Computer Vision can analyze visual data from financial markets and identify patterns, trends, and anomalies. | Enhanced surveillance and security, Automated document processing, Streamlined fraud detection |
Robotic Process Automation (RPA) | Robotic Process Automation (RPA) involves using software robots to automate mundane and repetitive tasks in business processes. | RPA automates manual, repetitive tasks in capital markets, such as data entry, document processing, and reconciliation. | Increased efficiency and accuracy, Improved compliance and error reduction, Enhanced customer service |
AI Making The Business Case
A Triage of Data, Algorithms, and Manual Processes
Capital markets are a great candidate for AI innovation and AI implementation. Given that they deal with data, algorithms, and labor-intensive manual workflows, AI can work to their advantage.
Let’s find out how each of these components adds to AI’s potential success for the capital markets.
Data As The New Oil
Data has become a strategic asset in the securities market, analogous to the economic value of oil. Just as oil powers industries, data fuels AI algorithms, enabling market participants to extract valuable insights and make informed decisions. The sheer volume of data generated from trade transactions, market feeds, and news sources, and provides scope for AI. AI can tap into this vast resource and uncover patterns, trends, and correlations that can drive trading strategies, risk management, and overall market performance.
With the proliferation of news articles, research reports, and social media discussions, unstructured data has become a valuable source of market sentiment. AI-powered sentiment analysis algorithms can comb through vast volumes of unstructured data, extracting insights on investor sentiment, public opinion, and market trends.
Algorithms, The Foundation of Automated Trades
The integration of AI with algorithmic trading opens up new frontiers for predictive analytics and trade optimization. AI algorithms can analyze vast volumes of historical trading data, market feeds, news sentiment, and social media chatter. These automated algorithms then generate predictive models that inform trading strategies for market participants. For instance, AI can enable algorithms to automatically adjust trading parameters based on real-time market conditions. This helps improve trade execution efficiency, leading to optimized trading outcomes/
Manual Processes and Automation
Manual data entry or data extraction from various sources often leads to errors, inconsistencies, and a lack of standardization. AI algorithms can automate data collection, ensuring high-quality information. Additionally, AI can streamline data cleansing and standardization, enhancing data accuracy. AI-powered algorithms can analyze complex trade data, compare transactions across multiple systems, and automatically identify discrepancies, facilitating speedy trade reconciliation. This has the potential to drastically reduce manual effort and processing time. What’s more – studies show that AI can automate up to 90% of trade reconciliation tasks.
By leveraging the convergence of data, algorithms, and human interaction, AI is driving innovation, enhancing decision-making capabilities, and bringing unprecedented efficiency to the securities market.
Exploring AI Use Cases for the Securities Market
A raft of regulations, data flowing across multiple systems, and highly volatile trades have led broker-dealers to turn to AI for addressing challenges as well as driving positive change across the entire trading lifecycle. We discuss 3 key areas.
- Redefining Customer Experience
- Innovating Investment Processes
- Driving Operational Efficiency
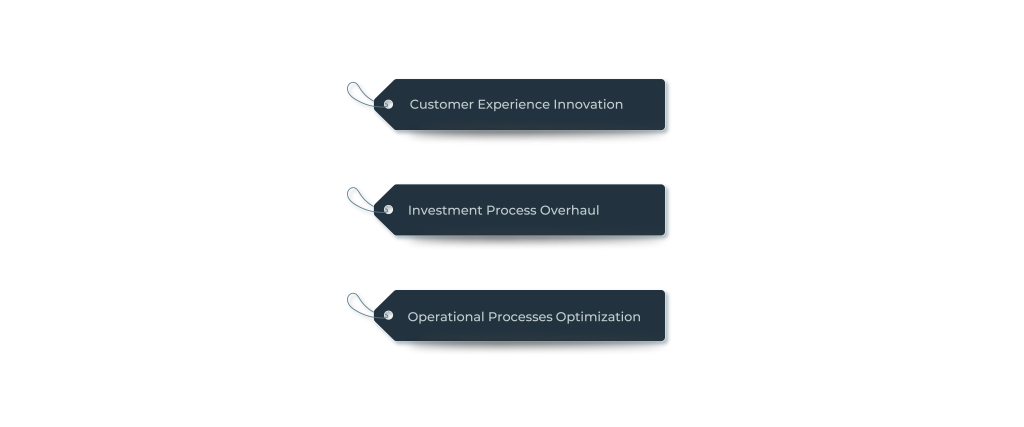
Redefining Customer Experience
Broker-dealers in the securities industry are leveraging AI to refine customer experience and enhance various aspects of their operations.
Virtual Assistants
Through AI-powered virtual assistants, broker-dealers are exploring the delivery of intelligent customer service. To help generate faster response times in addition to offering a personalized experience, virtual assistants can help in the following ways:
- Provide responses to basic customer inquiries related to account balances, portfolio holdings, and market data.
- Offer the ability to accept and process trade orders within certain limits.
A case in point is Capital One, an AI-first financial services firm leveraging AI’s potential for delivering superior customer experience. With its chatbot Eno, Capital One uses NLP and ML to answer customer queries, provide account information, and offer financial insights, enhancing the overall customer experience.
Email Followups
Currently, AI’s use case with customer experience is widely used to screen and classify incoming client emails, automatically responding to routine inquiries and routing complex ones to the appropriate personnel.
Customer Outreach
Broker-dealers are tapping AI to target outreach to customers and potential customers by analyzing their investing behaviors, website and mobile app footprints, past inquiries, and customer profiles. This allows them to proactively provide customized content like educational information, news, and research reports.
Customer Profiling
In brokerage account management, AI-based tools are utilized to provide customized, real-time customer information to individual brokers. This enables them to develop holistic customer profiles that incorporate a wide range of data sources, including customer assets, spending patterns, social media updates, browsing history, and past communications. This information helps brokers enhance customer relationships, provide better service and recommendations, and minimize customer attrition.
Innovating Investment Processes
Several companies are leading the way in implementing AI in the investment process. For instance, BlackRock, one of the world’s largest asset managers, utilizes machine learning algorithms to enhance its trading capabilities. Additionally, J.P. Morgan has developed an AI-powered research platform called LOXM, which assists traders in making faster and more informed decisions. Here are some prominent features driving innovation with investment processes.
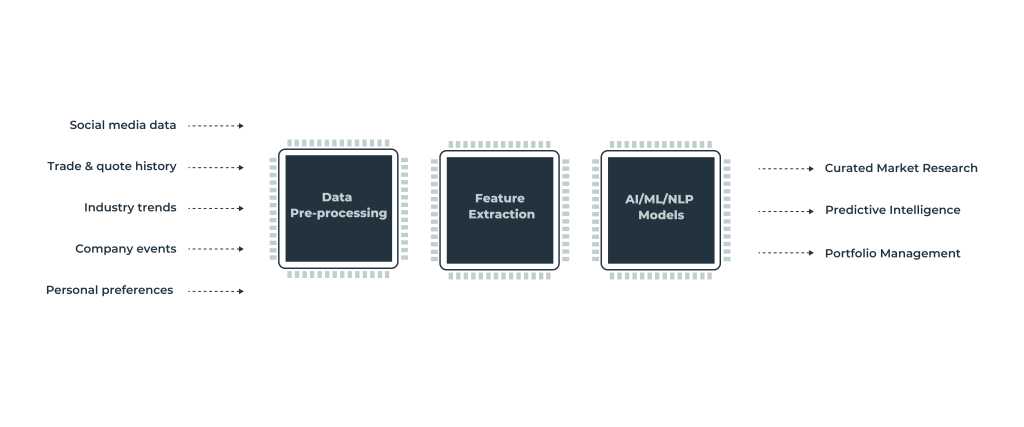
Curated Market Research
AI tools are being employed by broker-dealers to provide customers with curated market research.
- AI tools offer social media data and sentiment analysis on investment products and asset classes.
- By leveraging AI, broker-dealers can tailor investment suggestions to individual customers, offering personalized recommendations based on their unique circumstances.
- Akin to Netflix’s recommendations algorithm, a recommendation engine would help understand what data is most important to each individual (e.g. using page views to understand what was viewed by whom for how long), as well as suggesting closely related research (based on portfolio composition and trading behavior for example) to help them explore new sources of insight.
Predictive Intelligence
Proprietary data from investment banks can be used to understand the views and preferred opportunities of different investors. Analysis of unstructured data (e.g. old deals, total money raised, founding-team background etc.) using machine learning can generate indicators of future success. Increased speed of analysis using machine learning allows institutions to test correlations in a more automated way. This reduces the effort required to build models, freeing resources to do more qualitative analysis (e.g. interviews).
Algorithmic matching systems can support the creation of pitch books by determining the most important factors that investors consider and synthesizing key points, which will better identify and match deal prospects and deal opportunities.
Portfolio Management
AI applications are used within portfolio management to identify new patterns and predict potential price movements. These applications utilize vast amounts of data from sources such as social media and satellite imagery to uncover insights that may signal price movement. This information is then incorporated into investment strategies, aiming to generate alpha for the portfolio.
Driving Operational Efficiency
There is a growing emergence of AI use cases in post-trade settlement and reconciliation processes. Straight up, AI can replace manual reconciliation process with automation resulting in greater liquidity for investors and faster settlement time. Through the use of predictive analytics, AI tools can prepare brokerage firms toward a proactive regulatory stance. Here we discuss prominent use cases.
Compliance And Risk Management
In 2022, the SEC laid a sanction of US$6.4 billion as fines for non-compliance. As much as the rapid rise in the use of digital technology is freeing up manpower and streamlining complex workflows, it is also leaving room for unregulated trades. On top of this, data-privacy regulations make the scenario more complex. AI-based tools could help in avoiding regulatory intervention and penalties by red-flagging potentially fraudulent activities and settlement-failure risks.
Surveillance And Monitoring
AI-powered surveillance systems have the ability to analyze large volumes of data in real-time. This helps to significantly enhance the detection of market manipulation or insider trading. A 2020 KPMG whitepaper indicates a good 80% of their survey respondents acknowledge AI as improving the effectiveness and efficiency of their surveillance programs. Notable use cases include the application of ML algorithms to detect patterns of suspicious trading activities or abnormal market behaviors.
Detection of Settlement Failures
Settlement failures in capital markets can have significant repercussions, leading to delayed transactions and increased costs. However, AI-powered systems have exhibited remarkable capabilities in reducing settlement failures.
DTCC’s Exception Manager utilizes AI algorithms to identify potential settlement failures, alerting market participants and enabling them to rectify errors proactively. This solution has revolutionized the settlement process, ensuring enhanced operational efficiency and minimizing risks.
Liquidity And Cash Management
Accurate liquidity and cash management are crucial for overall stability in capital markets. AI technologies play a pivotal role in optimizing liquidity positions and streamlining cash management processes. Statistical data indicates that AI-driven cash management systems have reduced idle cash holdings by up to 33%, allowing financial institutions to unlock significant capital for investments and business growth. J.P. Morgan’s Liink project leverages AI and blockchain technology to revolutionize cash management processes. By providing real-time visibility and predictive analytics, Liink offers unparalleled efficiency in cash allocation, resulting in substantial cost savings and improved liquidity positions.
Credit Risk Management
Traditional credit risk management techniques often fall short in accurately assessing complex credit portfolios. AI-powered systems, on the other hand, can analyze vast amounts of data, leading to more accurate risk evaluations and reduced credit losses. A recent survey by Accenture revealed that companies using AI in their credit risk models experienced a 25% decrease in non-performing loans. Moody’s Analytics RiskBench employs AI algorithms to enhance credit risk assessments. By analyzing historical data and incorporating real-time market insights, RiskBench provides financial institutions with comprehensive risk profiles, enabling them to make informed credit decisions and mitigate potential losses.
Beefing up Cybersecurity
With the growing threat of cyber-attacks, safeguarding financial systems becomes paramount. AI-driven cybersecurity solutions have demonstrated exceptional capabilities in detecting, preventing, and mitigating security breaches. A survey conducted by Capgemini found that AI-powered cybersecurity systems detected 94% of cyber breaches, significantly outperforming traditional methods. Darktrace’s AI-powered cybersecurity platform leverages machine learning to detect anomalous patterns, swiftly identifying and neutralizing potential threats. Its real-time threat visualization allows organizations to proactively defend their systems and data against cyber-attacks.
Next Steps
To make the move to AI and sustain a successful AI journey, broker-dealers should start with technology familiarization workshops and interviews with business stakeholders to identify their needs and how AI can support them. Also, add an initial risk assessment for every opportunity identified in order to prepare for long-term strategic goals.
Focusing on pilot projects with specific use cases is a great idea to test success at scale.
For instance, developing AI-driven chatbots or virtual assistants for customer support can improve response times and enhance the overall customer experience.
Implementing AI for the capital markets frequently requires significant structure, software, and mindset investments. Conducting a thorough cost-benefit analysis is pivotal to ensure that the implicit benefits outweigh the challenges.
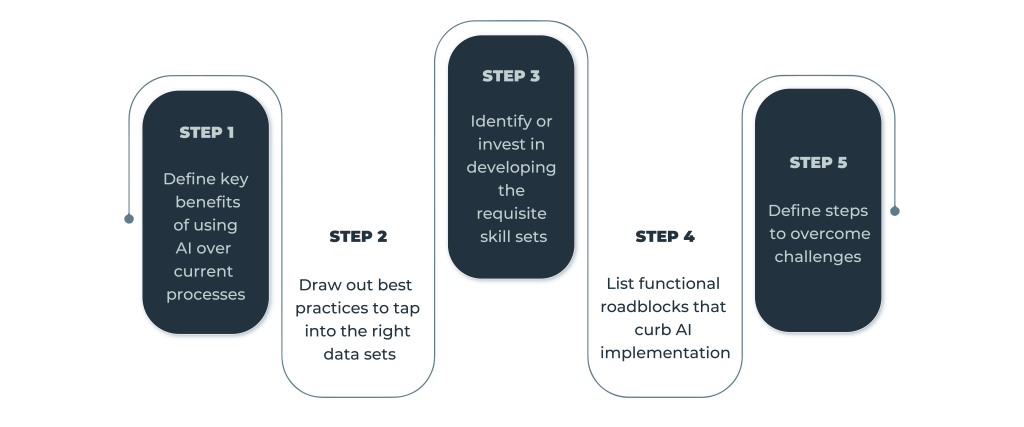
Outlined below are a few considerations that can help organizations roll out AI within their trading lifecycle:
- Conduct AI discovery workshops: Conduct workshops to acquaint your firm with AI that includes key decision-makers.
- Find the right implementation team: Identify a lean team with proven abilities to get things done in the organization and accelerate goals.
- Build technology architecture: Identify and close capability gaps, and develop supporting technology architecture and infrastructure (cloud and microservices) based on the processing power required.
- Identify data: Gather the right data spread across silos of cross-functional groups and legacy systems and eliminate inconsistencies to provide accurate data sets.
- Practice incremental deployment: Start small, prioritize desired tactics, identify select use cases to prove efficiency and value, gather feedback, and expand gradually.
- Scale up: Use an agile, iterative piloting approach to move from strategy to prototyping as quickly as possible. “Fail-learn fast” and achieve rapid results.
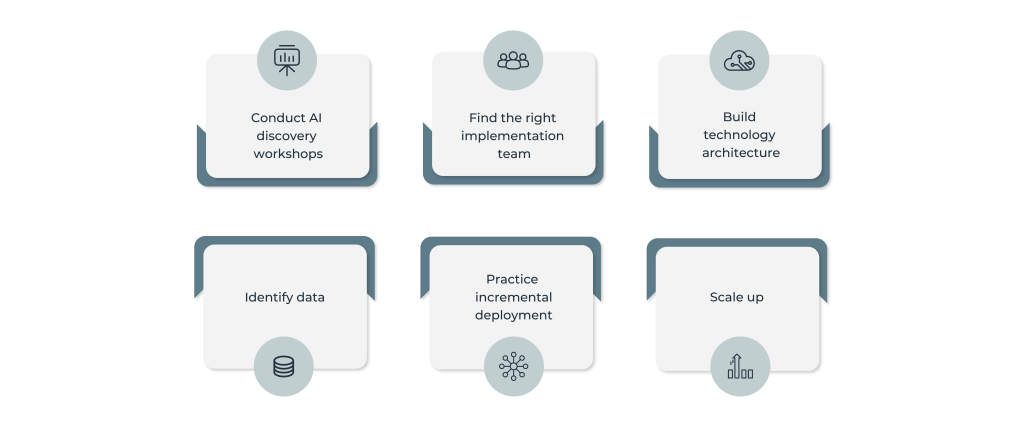
Wrapping up
To drive real change, it’s crucial to think of AI beyond the mere automation of repeatable processes.
Think of it as a technology modernization drive to be nimble for future advancements.
For capital markets, AI can accelerate the development of innovative products and IP, which are key areas in the digital age.
That said, choosing a trusted technology partner can unlock AI-driven value at scale. At Ionixx, we pride ourselves on being your delivery partner; seamlessly combining our domain financial services expertise with our experience in delivering value through AI and other state-of-the-art technologies. Get in touch.