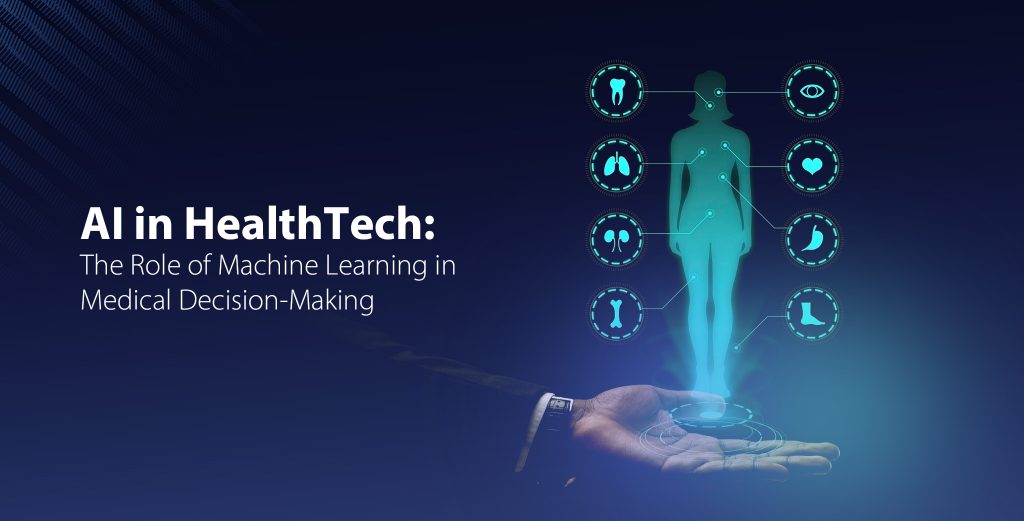
The term AI has become ubiquitous in the first half of 2023, with every industry hopping on the bandwagon of ChatGPT and GPT-4. Healthcare is no different, though we have had 10+ years of “AI” and Machine Learning Algorithms, the new Transformer architecture has pushed AI into uncharted territory far earlier than experts had predicted!
As we delve deeper into the realm of AI in healthcare, we will explore its transformative impact on telemedicine, medical decision-making, and the building blocks that make these advancements possible.
AI, Machine Learning and Deep Learning: The Basics
Understanding the foundations of AI and its subfields, Machine Learning (ML) and Deep Learning (DL), is crucial for appreciating its impact on medical decision-making.
Machine Learning:
A subset of AI, ML involves the development of algorithms that improve through experience by learning from data. ML models can be trained to recognize patterns, make predictions, and adapt their behavior as they process new data. In the context of telemedicine, ML algorithms can analyze patient data, medical images, and other relevant information to assist healthcare providers in diagnosing diseases, personalizing treatments, and monitoring patient health.
Deep Learning:
Deep Learning is a more advanced subset of ML that uses artificial neural networks to model and solve complex problems. These neural networks are inspired by the structure and function of the human brain, allowing them to process and interpret data hierarchically. Deep learning models can handle large amounts of data, including unstructured data like images and text, making them highly suitable for tasks such as medical image analysis, natural language processing, and advanced predictive analytics.
ML in Stroke Detection: Improving Patient Outcomes
ML algorithms are capable of analyzing vast amounts of medical data, from sources such as electronic health records (EHRs), medical imaging, and lab results, to identify patterns and trends.
One example is in stroke detection. There are several methods physicians can use to detect strokes (NIHSS, CHA2DS2-VASc, ABCD2), and AI-driven Stroke detection algorithms are being continuously developed using these scoring systems using real-time data from EHRs and other sources.
Where Do The Gaps Lie?
Despite the promise of these AI-driven tools, implementation so far has been far from perfect. A significant issue faced in clinical settings is alert fatigue, wherein healthcare providers are inundated with a multitude of notifications and alerts, many of which may be false positives or of low clinical relevance. Under the guise of safety, these alerts can lead to desensitization and reduced responsiveness to genuinely critical notifications.
To harness the full potential of ML for stroke recognition, it is crucial to address the issue of alert fatigue by refining the algorithms to minimize false positives and prioritize alerts based on their clinical significance. This should involve fine-tuning the model parameters, incorporating feedback from healthcare professionals, and using deep learning to improve the accuracy and relevance of the generated alerts. By doing so, the implementation of stroke recognition algorithms can become more effective, empowering healthcare providers to deliver better care and potentially save lives.
Remote Patient Monitoring And Predictive Analytics: Proactive Care
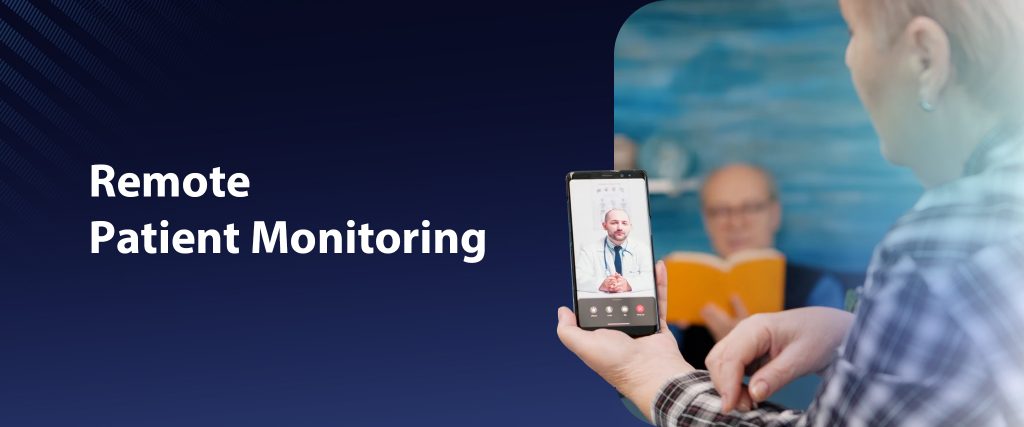
Telemedicine relies heavily on remote patient monitoring, which is greatly enhanced by ML algorithms. These algorithms can analyze data from wearable devices, mobile apps, and other monitoring tools to detect health changes, predict potential complications, and give alerts to the appropriate professional. This proactive approach to patient care (aka preventative care) can enable timely interventions, prevent complications, and improve overall patient satisfaction.
My adage to judge all emerging health technology is: “Information without Intervention is useless”.
A lot of this data is in heaps of unusable mountains that the physician cannot access at the point of care. What we need to focus on is getting accurate, actionable information to the physician when they are able to alter the course of treatment.
Personalized Treatment Planning and Recommendations: Optimizing Patient Outcomes
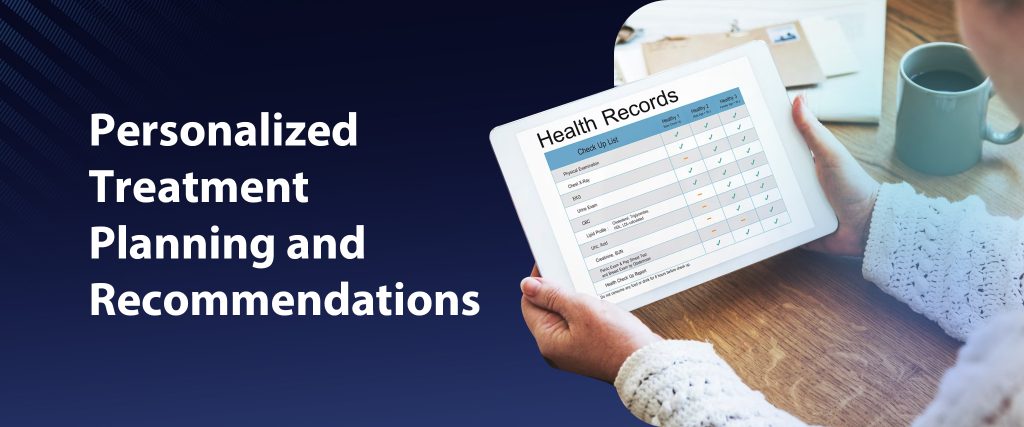
ML algorithms can assist physicians in developing personalized treatment plans for patients by analyzing their medical history, genetic factors, and other relevant data. This approach can help identify the most effective treatment options, minimize adverse effects, and optimize patient outcomes.
Again, first accuracy of this data must be established, then presented to the physician in a manner they can easily review at the point of care. ML can offer real-time decision support by supporting appropriate interventions or adjustments to treatment plans based on genetic factors.
Today it is already cheaper and faster than ever to do a genetic analysis from a cheek swab, taking only 20 minutes to sequence and glean insights – this swab can even be done in the waiting room with results in your doctor’s chart by the time your appointment begins!
Where we are Headed – Challenges And Opportunities for AI-powered Healthcare
The AI revolution has barely begun, yet it is already transforming the way healthcare is delivered from multiple angles. Machine learning algorithms will undoubtedly play an increasingly pivotal role in enhancing medical decision-making. By incorporating ML algorithms in diagnosis, treatment planning, remote patient monitoring, and telemedicine, providers can offer more accurate, personalized, and proactive care to patients.
However, it is crucial to address the challenges and ensure that AI-driven medical decision-making tools are developed and implemented within optimal workflows. We need technology that supports physicians in their work rather than impeding their progress or consuming more time. By striking the right balance and focusing on the ultimate goal of improving patient care and outcomes, we can harness the full potential of AI and machine learning in healthcare, shaping a better future for patients and providers alike.